Businesses thrive or die by the quality of their data. In this article I will describe three examples of good, bad and ugly approaches to data management, with some lessons learnt at the end. These have all come up in the course of our work, but I have made some changes to protect against identification of any specific companies.
1. The Ugly
At this time of year, we are often asked to support clients with their annual tax reporting processes for FATCA and CRS. Due to the limitations of their accounting system, this trust company needed to pull data out of their system to perform some computations, before they could generate the XML tax reports.
We were brought in at the end of the process to convert the data to XML and upload to the reporting system.
This should have been a relatively simple process of taking the Excel based data, saving as a txt file and then uploading. If only life was that simple…
The source data had been exported from the accounting system into multiple spreadsheets with key data missing.
A lot of unnecessary manual data manipulation had taken several people weeks to prepare in Excel, before I spent a day of checking the integrity of the uploads before converting to XML.
Most of this work could have been avoided with minimal investment in data automation tools – which I will come back to at the end.
This is a business that, like many, is struggling to find staff in a difficult labour market, but still spends significant time on non-value add data manipulation work that can’t be charged for and could be avoided with a bit of extra effort.
2. The Bad

This company has engaged us to help migrate from a legacy client administration system to a new cloud based system.
For various reasons it has proven difficult to get our hands on the source data, which needs to be reformatted before uploading to the new system. This is a fairly standard ETL process (Extract, Transform and Load) that is part of most system implementation projects.
Rather than give us access to the source database the client has decided to manually extract the data and provide it to us in text files.
What’s bad about that? We have the data and have been saved the job of doing the extraction. Shouldn’t we be happy?
The problem is the extracts were done over a period of a week whilst the system was in use, meaning some tables will have changed and data integrity compromised.
Data migrations are an iterative process and we will need to perform at least 3 more iterations before we go live in the new system. With each iteration we will need a fresh copy of the data, requiring the same manual effort.
What makes this really bad is that we have some state of the art data analytic tools that we haven’t been able to use because we aren’t being permitted to access the source database.
3. The Good
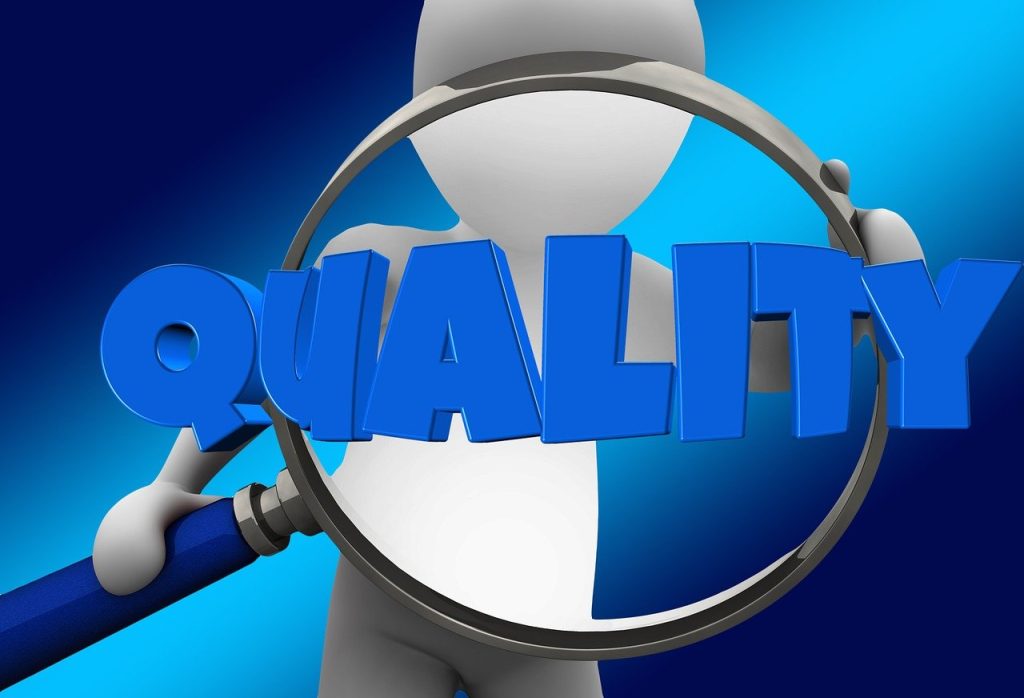
This is another system migration, this time from a 25yr old Microsoft Access database. This had the potential to be both the bad and ugly candidate for these examples, but I was pleasantly surprised and now hold it out as an example of best practice.
Although creaking and clearly suffering from all the issues you’d expect of a system of this age, the data itself was of excellent quality. The business had implemented strict controls over data input to ensure data integrity was maintained.
We have access to the database and can easily perform the ETL and this has the hallmarks of being a good data migration project.
Lessons learnt
- Whether you are using Microsoft Access or more sophisticated databases, it is the people who manage the data that have a much bigger impact on its quality than the technology itself
- One of the biggest barriers to good data management is the ease of use and availability of Microsoft Excel. Very quickly a series of one-off spreadsheets can easily become business critical, with no controls around their creation or upkeep.
- Resistance to change and people protecting their self-interest can be a significant barrier to progress in good data management.

Finally, getting back to the technology of data management and data-driven decision making, a tool that can help get around many of the issues described above is Alteryx.
I’ve written about Alteryx before in this blog post on the importance of data quality. You can read more about it here.
Over half our team is now Alteryx qualified and I achieved my Core qualification this week – on one of Digital Jersey’s free course run by Continuum, one of Europe’s leading Alteryx partners. Hence my focus today on data management.
Seeing how easy Alteryx is to use and get great repeatable results means I take much more notice when I see bad and ugly data practices.
If you need help with your data, want to see how the power of Alteryx could transform your decision making, or want to talk through some of your people challenges around maintaining good data, then give me a call or use the Contact Us form. I’ll get back to you to arrange a time to discuss.